Do you still think availing email addresses and phone numbers of your prospects are enough to close a profitable deal? If yes, then you are wrong.
Sales happen when your prospects engage with you. And they engage when they resonate with whatever you say.
To make them resonate with your message you need a complete understanding of them, like demographic, firmographic, technographic, and many such details of your prospects.
According to a report, 66% of customers claimed that they want brands to understand their unique needs and expectations.
But how do you get such details? That’s when data enrichment best practices comes into the picture.
By enriching your existing data with external sources, you can gain valuable insights and improve targeting for personalized campaigns. This helps you to improve engagement, generate leads and convert them into your customers.
So, if you want to take your marketing game to the next level, keep reading as we explore the data enrichment best practices that are key to successful marketing campaigns!
What is Data Enrichment?
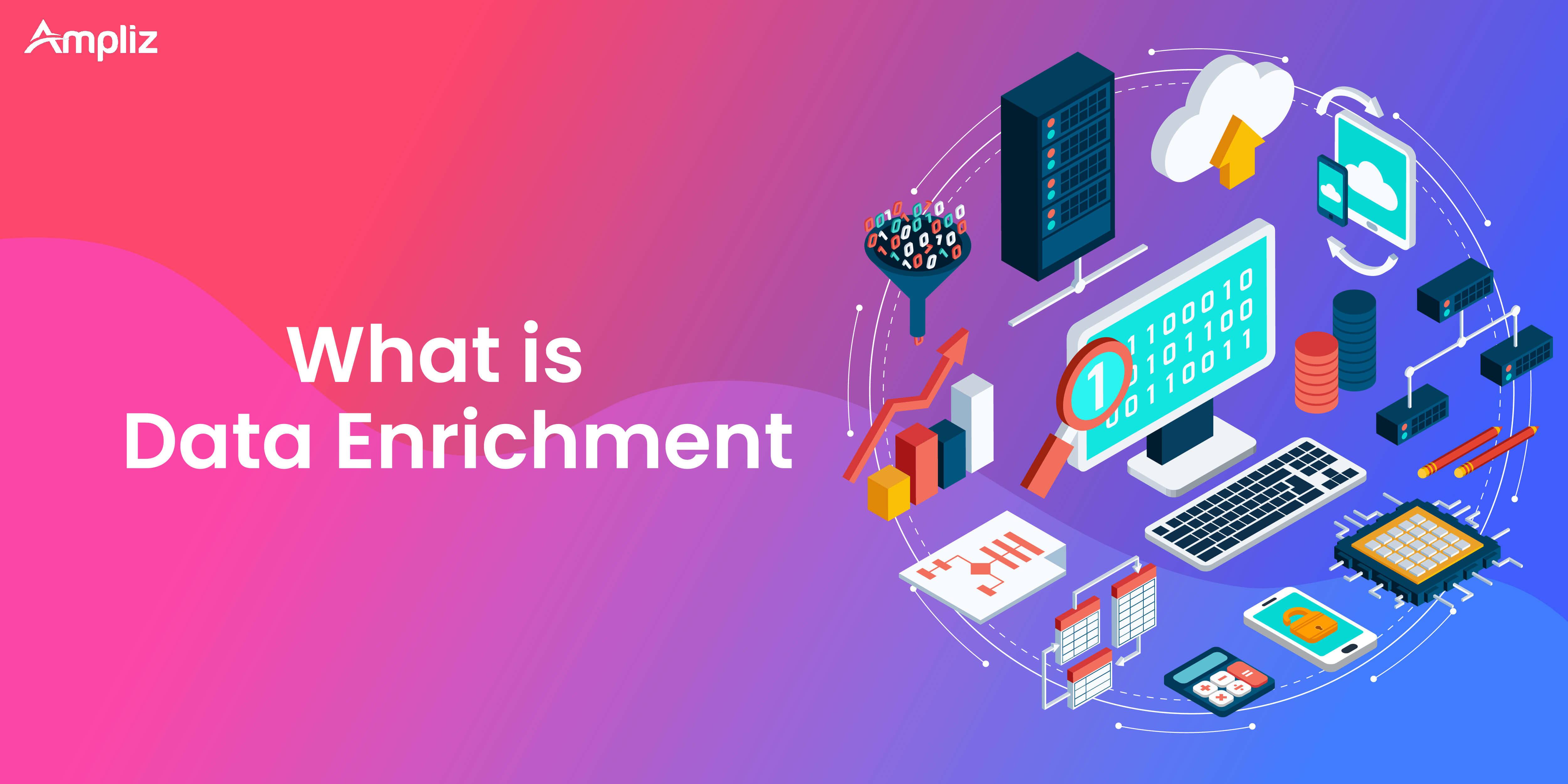
Data enrichment is the process of adding additional data to an existing dataset in order to improve its quality or value.
This can be done through a variety of means, such as adding new data points, imputing missing values, or deriving new features from existing data.
As an example of data enrichment, let’s say you run an e-commerce business selling sporting goods online.
You have a list of customer names and email addresses in your database but don’t know much about them beyond this basic information.
With data enrichment techniques, you can add more details such as age range, gender, location (city/state/country), purchasing history (what items they’ve bought previously), website browsing behavior (what pages they’ve visited on your site), social media activity (likes/comments/shares) – to name just a few examples.
By adding these new data points, imputing missing values, or deriving new features from existing data, marketers can significantly improve the quality and value of their datasets.
This, in turn, leads to more effective campaigns with effective personalization. This helps them build a strong relationship with their customers and improve their ROI.
Benefits of Data Enrichment
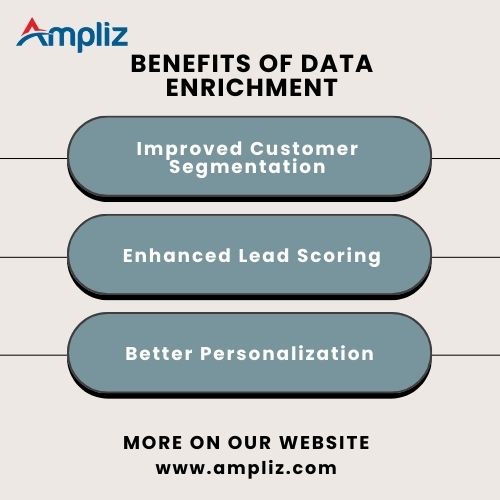
Data enrichment process helps you to prioritize your customer over everything else and make your marketing efforts completely customer-centric.
This helps you to improve your customer segmentation, enhance lead scoring, improve your personalization, and so on.
Let’s see these benefits of data enrichment in brief.
1. Improved Customer Segmentation
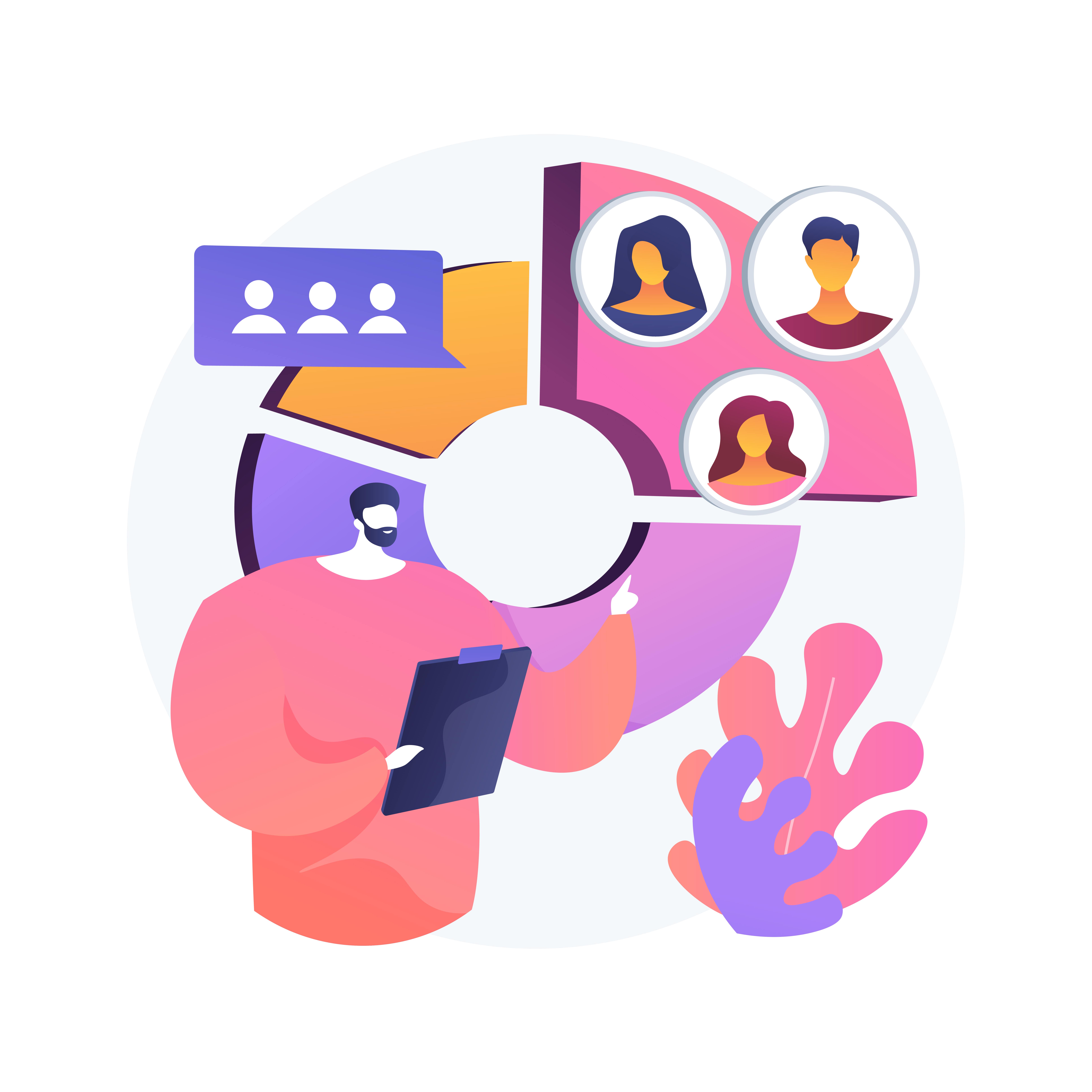
You can effectively segment your customers by enriching your data with the details like last purchase, the demographic, psychographics, and the amount spent.
This allows you to create targeted email marketing campaigns that resonate with different customer groups.
Data enrichment techniques plays a crucial role in helping businesses create effective segments by providing additional attributes that can be used for classification.
For example, if a you have basic customer information such as name and address but lack details about their buying habits or interests, you can use third-party sources like social media platforms or purchase history databases to enrich the available data with this missing information.
By using enriched data strategy in segmentation analysis tools like clustering algorithms or decision trees, you can gain a better understanding of your target audience’s unique characteristics.
You can identify patterns within different segments that help them develop focused marketing campaigns tailored to each group’s interests and needs.
Data Enrichment strategies helps in Data Segmentation through:
1) Providing additional attributes that aid in classification
2) Giving deeper insights into consumer behaviors & preferences
3) Facilitating personalization based on segmented groups
2. Enhanced Lead Scoring
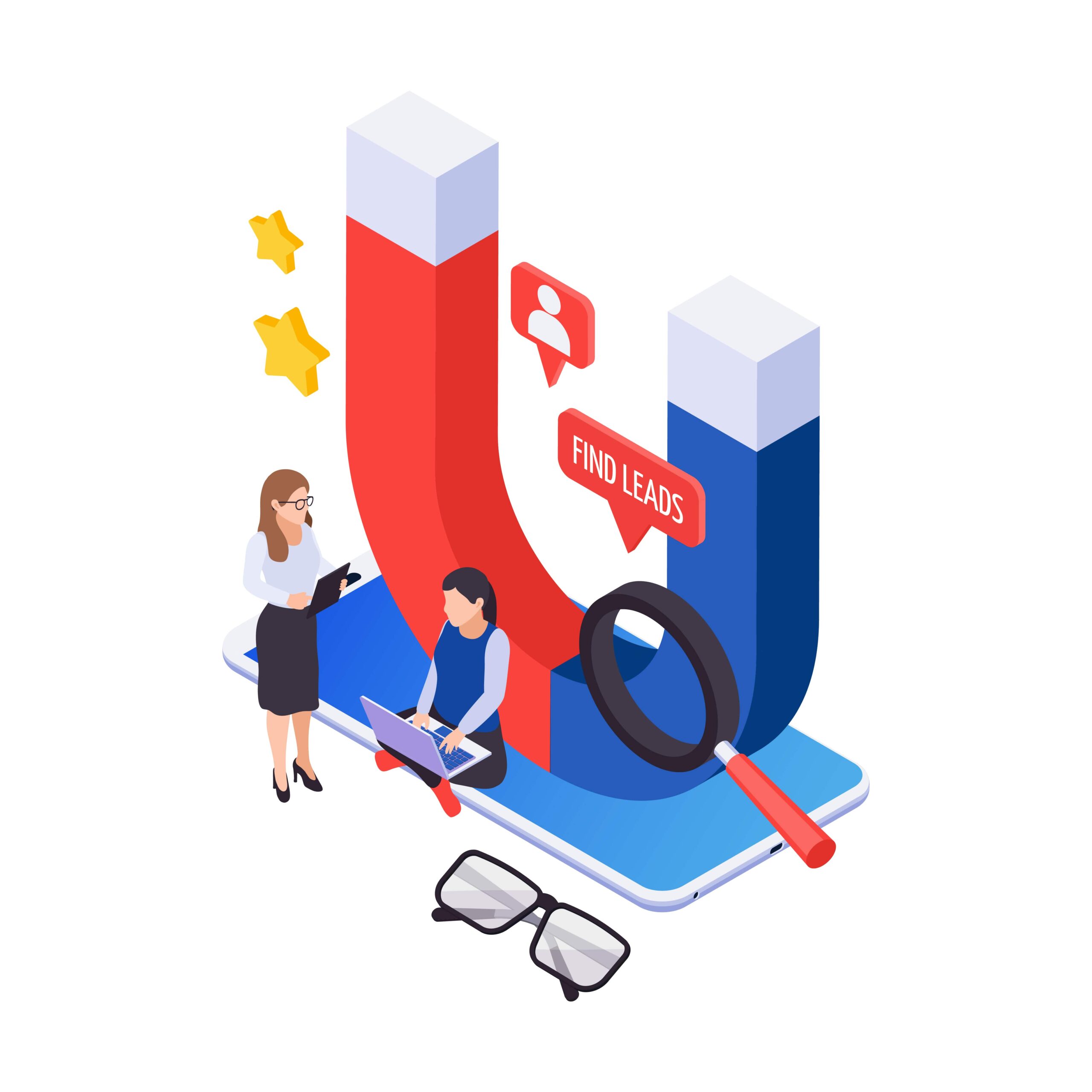
In the context of lead scoring, data enrichment best practices plays a critical role in improving the accuracy and effectiveness of lead scoring models.
By enriching the available data about leads with additional information such as job titles, industry affiliations, social media profiles, and company size or revenue, you can get a more complete picture of their potential customers.
This added insight helps to identify which leads are most likely to become paying customers and prioritize them accordingly.
Marketing strategies for data enrichment also allows for better identification of high-value accounts that might otherwise be overlooked.
By identifying factors that indicate strong buying intent – such as recent website visits or engagement with marketing materials – enriched datasets allow marketers to pinpoint those companies worth pursuing more aggressively.
Ultimately, by leveraging enriched data sets in combination with advanced algorithms for lead scoring – such as machine learning models you can create sophisticated systems for identifying which leads are most likely to convert into paying customers.
These insights enable sales teams to focus on high-value prospects while avoiding wasting time chasing after low-potential opportunities.
3. Better Personalization
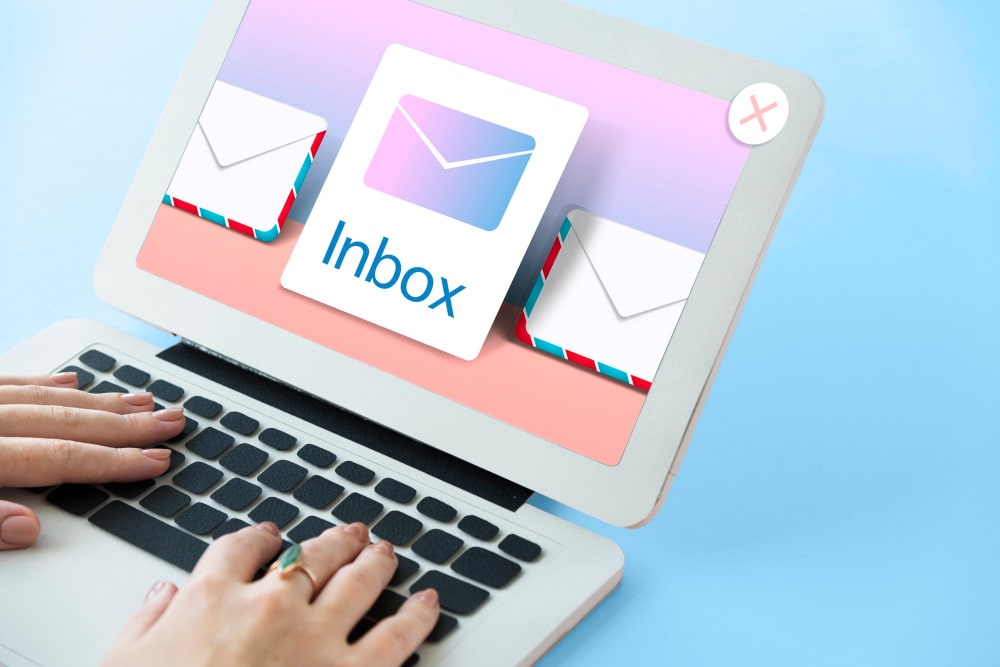
By enriching customer data, you can create highly targeted and personalized marketing campaigns that resonate with individual consumers.
For example, if you have access to customer purchase history through enriched data sources, you can use this information to suggest complementary products or services based on past purchases.
Data enrichment best practices also enables you to segment your audience into smaller groups based on common characteristics.
This approach allows for even greater levels of personalization by creating tailored messages for specific segments.
For instance, if an online retailer uses enriched data to identify high-income shoppers who prefer luxury goods over budget items, they can send promotional emails featuring premium products that appeal specifically to those shoppers.
Moreover, the ability to add new details about customers using external databases or third-party applications further enriches existing data sets.
By incorporating social media analytics activity logs and other publicly available information into customer profiles via algorithms or machine learning models that automatically update them in real-time for accuracy purposes; businesses gain deeper insights into what drives consumer behavior patterns and how best they could serve their needs accordingly.
Data enrichment enhances personalized experiences by:
1) Creating a better understanding of customers’ preferences
2) Segmenting audiences into smaller groups
3) Providing more comprehensive insights
4) Incorporating external sources like social media activity logs
Therefore leveraging these benefits of data enrichment improves personalization which leads towards stronger relationships between businesses & customers ultimately driving revenue growth!
Data Enrichment Best Practices 2023
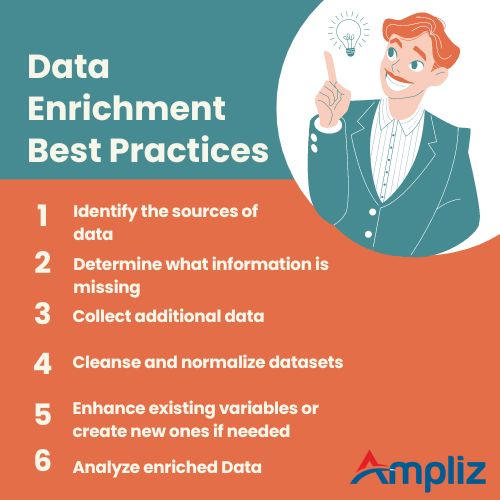
Data enrichment best practices are essential for businesses wanting to gain valuable insights from their customer data and give themselves an edge over competitors.
They should include ensuring the accuracy of the data, regularly updating it, using a holistic approach where possible, as well as leveraging automation tools for faster results.
With these tips in mind, companies will have all they need to ensure success with their marketing campaigns.
Enriching collected data is crucial for businesses to gain valuable insights that can drive decision-making, but it requires a systematic approach. Here are some steps you can take:
1. Identify the sources of data:
Start by listing all the potential sources of data, such as customer feedback forms, customer feedback tools, social media platforms, web analytics tools, and third-party databases.
2. Determine what information is missing
Once you have listed all the possible sources of data, identify which information is missing from the existing dataset that could help you achieve your business objectives.
3. Collect additional data
Depending on what information is lacking in your dataset or what questions you need answered to make informed decisions; decide where to collect more relevant data and how to do that efficiently.
4. Cleanse and normalize datasets
Before enriching collected data with new variables or dimensions ensure any issues with quality are fixed like duplicate removals etc.
5. Enhance existing variables or create new ones if needed
This step involves adding more context by introducing additional fields/data points based on both internal & external factors relative to analyzed dataset
6. Analyze enriched Data:
Finally in the data enrichment best practices, use visualization techniques like charts/graphs/pivot tables/ dashboarding solutions with sophisticated Business Intelligence tools so decision makers can easily grasp most important insights hidden behind vast amounts of enriched collected raw-data.
By following these steps, businesses can effectively enrich their collected datasets and turn them into actionable insights that inform better decision-making processes across organizations’ departments. Additionally, incorporating incremental change data capture (CDC) replication can further enhance the data enrichment process. This technique allows businesses to continuously capture and replicate only the changes made to the dataset, minimizing processing time and ensuring that the enriched data remains up-to-date and accurate in near real-time.
Conclusion
Data enrichment best practices are essential for businesses wanting to gain valuable insights from their customer data and give themselves an edge over competitors.
They should include ensuring the accuracy of the data, regularly updating it, using a holistic approach where possible, as well as leveraging automation tools for faster results. With these tips in mind, companies will have all they need to ensure success with their marketing campaigns.
FAQ: Data Enrichment Best Practices
What are the Steps of Data Enrichment?
Data enrichment is the process of adding structure, context, and additional information to existing datasets.
The steps of data enrichment can be broken down into four stages:
identifying data sources, integrating data, cleansing and normalizing the data, and finally enriching the data.
Identifying the right data sources is essential in order to add more value to your dataset.
Next, you’ll need to integrate those sources with your current dataset. This often requires complex ETL methods.
After that comes the cleaning and normalization step, which removes inconsistencies and makes sure everything is consistent across all sources.
Finally comes the actual enrichment phase – this is where you get to add relevant information from outside sources that can add a lot of value to your dataset.
What is data enrichment techniques?
Data enrichment techniques are approaches used to enhance the quality of data by adding additional information that can be used to improve the accuracy, completeness, and usefulness of the data.
This may include gathering information from external sources, integrating data from different sources, and cleansing or deduplicating existing data sets.
By doing so, businesses can gain valuable insights and better understand their customers’ needs.
Why data enrichment is important?
Data enrichment is important because it helps you understand your customer’s needs and preferences, so you can create more personalized experiences.
It also helps you better understand market trends and anticipate changes in customer behavior. Data enrichment provides the necessary context to make smarter decisions faster and with greater accuracy.
By enriching your data, you enable better analytics that provide actionable insights for your business.